Behind the Algorithm
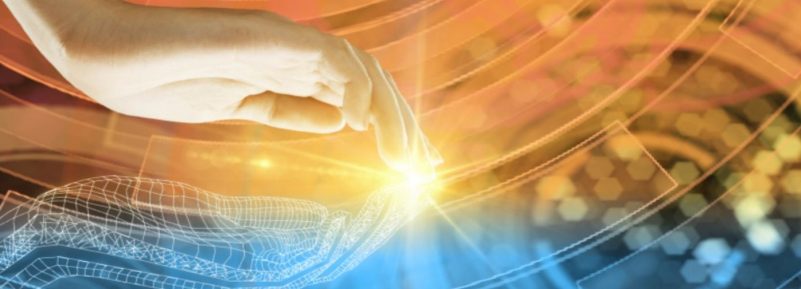
Whether it is chatbots for customer interaction, neural networks for identifying the patterns tucked in the data or machine learning for improving decision-making, AI is driving the transformation of business and value creation in new directions. According to a 2017 study from Cowen and Company, 81% of IT leaders are currently investing in or planning to invest in AI. Angel.co has counted 2,200 AI startups, half of which have emerged in the last two years. By 2019, those startups and others like them will "overtake Amazon, Google, IBM and Microsoft in driving the [AI] economy with disruptive business solutions," according to Gartner.
Few people are as well-positioned as technologists to understand how artificial intelligence is changing the world. After all, IT professionals are accustomed to exploring new automation technologies with the potential for transforming how work gets done.
Most forms of automation focus on performing repetitive, menial tasks. AI's sweet spot, on the other hand, is in automating tasks that can now be performed by algorithms using access to relevant data. Machine learning, a subset of AI, is an approach to make algorithms "smarter" as they “learn” from more examples. That’s one of the reasons why big data and AI seem to be evolving together. "With more examples, we can get better algorithms and better prediction accuracy," explains Kendall Giles, an assistant professor of practice in the Department of Electrical and Computer Engineering at Virginia Tech and a faculty member in the university's online Master of Information Technology (MIT) program.
The use of big data and machine learning is just one of the Virginia Tech MIT course concentrations available to students. Those pursuing this degree will position themselves to be in front of a wave of professional demand, with job titles such as:
- Machine learning engineer
- Data scientist
- Research scientist
- R&D engineer
- Business intelligence developer
- Computer vision engineer
Demand for AI specialists
"It is really not a far stretch to claim that every IT professional today must have a working understanding of data science to be successful," says Naren Ramakrishnan, the Thomas L. Phillips Professor of Engineering at Virginia Tech and director of its Discovery Analytics Center. "Seven out of 10 organizations have already invested or plan to invest in big data," he notes. "Every type of IT practitioner, whether systems or applications, will be impacted by these trends."
In fact, adds Giles, "There's a huge shortage in finding people with these skills, who know how to communicate with the computer, who understand technology and who can process data and enjoy doing that." According to the New York Times, AI specialists can demand compensation of between $300,000 and $500,000, including salary and company stock, with just a few years of experience. Why such sizable pay? Big companies are driving "a lot of the dollars," Giles says. "The Googles and Facebooks and Microsofts are able to hire and pay big bucks."
The human element
As part of the MIT program, Ramakrishnan teaches the popular course Machine Learning with Big Data. The course emphasizes how participants can use their own subject-matter expertise.
"In the machine learning paradigm, you have to learn about how computers can be trained to do certain tasks. If you're going to work in Amazon’s Whole Foods Market division, you might be thinking of training a computer to recognize vegetables. If you're going to be working in a bank, you have to train an algorithm to be good at understanding financial transactions and loans," he says. "In a sense, you become the human element — the trainer, if you will — behind the algorithm."
AI vs. machine learning
Artificial intelligence is an umbrella term used to describe the science of making computers act in intelligent ways. It includes technologies such as machine learning, natural language processing and speech recognition, among others.
Machine learning refers to algorithms that can constantly improve, either by:
- a human “teaching” it through continual feeding of new content, data and examples
- the algorithm learning through trial and error
In the course, Ramakrishnan uses machine learning examples from his collaborations with companies, agencies and other organizations. For example, a partnership between the Discovery Analytics Center and the Washington Post resulted in the creation of a system to help the publisher predict the popularity of its articles, sometimes even before they're published. A week of class is used to explore that collaboration: what data was used, how the project unfolded, how the model was developed and evaluated, and how it was finally applied. "That's of great interest," he says. "A lot of people learn machine learning as an academic curiosity, but very few people know how it's actually deployed in practice."
Such practical insights are an important part of the training students get, Ramakrishnan says. As another example, he cites the issue of model drift. "One thing that happens when you deploy machine learning is it appears to work great, but then over time life changes, and the model tends to drift and not stay in tune with reality. You need to start updating your model — but how and how frequently should you update your model?" he says. "We talk about these issues of keeping the model current — making sure your predictions and recommendations are still valid — and how you can do that at scale."
Something that’s interesting to Ramakrishnan is the diverse backgrounds of his students. No matter what industry they are in or intend to work in, everybody has a use for what they learn in this course, he says. That's useful when it's time to work on a team project. Students divide into groups and use virtual meetings to develop a problem involving an interesting data set, either public or proprietary. The goal is to develop a predictive application, algorithm or task that makes sense for that particular domain.
The goal of the MIT program’s new courses is to provide an advantage to students pursuing a graduate degree in technology. "There are a lot of entry points into machine learning," Ramakrishnan says. "Machine learning is almost like a Swiss Army knife. You never know which tool you'll need, but they're all useful somewhere."
The lure of AI and big data
Swati Gupta has worked at a financial services firm for more than a decade, most recently as director of software development in charge of in-house applications. But she feels like she's hit a plateau in her career. Gupta knew that making the leap from her current position to a higher role, such as CTO or CIO, would require her to augment her MBA with a master's degree in technology to solidify her standing in the field.
Gupta determined that an online degree would give her the flexibility she needed as a working professional and parent. "It doesn't limit you to certain times, and you can work on your own schedule," she says.
She was drawn to Virginia Tech’s Master of Information Technology for its variety of courses. In fall 2017, Gupta began her studies with a concentration on big data. Because of her background in programming, she was able to dive right into one module on machine learning with big data and another on cybersecurity, both applicable to her current work.
Now, Gupta participates in weekly video lectures, discussion forums, live meetings, assignments and group projects. The course with Professor Ramakrishnan had her working with a dataset of over 200,000 records to apply the algorithms they'd been studying in class to predict which genres that music companies or recording studios should invest in based on playlist activity. She says that type of problem, which uses regression modeling, can apply to financial services to determine which stocks are moving in the right direction — or not.
Even though Gupta has been at her studies just a few months, she already feels more confident in her current position because she can talk about the tech that permeates discussions in her company from a place of understanding.
"Virginia Tech's program is really beneficial for professionals like me who have been in the industry and are looking for cutting-edge knowledge in areas such as AI, machine learning, social media analytics and cybersecurity," she says. “I've attempted to learn these subjects through short online courses, but the graduate level coursework, along with the ongoing opportunities to collaborate online with my VT-MIT peers and professors, has provided a hands-on understanding of these subjects.”