Follow the Data
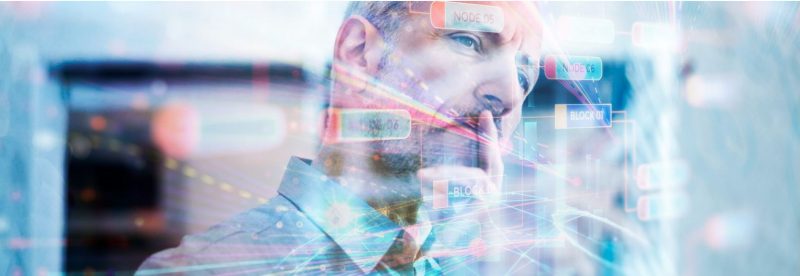
Some problems in the world like human trafficking look intractable. Then there are the everyday problems: figuring out how much inventory to deliver given the weather forecast, or what the best type of intervention would be to keep a student in college or how to identify just the right cocktail of treatments to address an individual’s specific cancer. Ralph Badinelli, a professor at Pamplin College of Business at Virginia Tech, says data analytics can help identify solutions for such problems and enrich decision-making.
Badinelli teaches courses in the Decision Support Systems module of the Online Master of Information Technology program at Virginia Tech. One course he teaches on cognitive computing explores the use of IBM’s Watson, the high-performance computing platform that combines artificial intelligence and data analytics. One way it’s used is to support the decision-making of oncologists.
“You would like an oncologist to be up to date on all of the latest research in chemotherapies, which means [he or she] would have to read literally thousands and thousands of research articles in journals all over the world,” says Badinelli. “Watson has read all those articles, so the oncologist can go to Watson and say, ‘Here’s the kind of patient I’m dealing with. What do you recommend?’ And Watson can come back with a recommendation for a course of therapy.” More than that, he adds, Watson can provide justification for the recommendation by referencing the literature -- those many journal articles -- it has “ingested” to extract that answer.
Watson, Siri and DSS
Watson is one dramatic example of cognitive computing. Apple’s Siri, Google’s DeepMind and Microsoft’s Cortana are other examples. Cognitive computing is a subset of a broader category known as “decision support systems” (DSS). These are systems that construct mathematical models of a decision.
When you hear about the use of business analytics to figure out what ad to serve up next on YouTube or determine the right price for a Lyft ride, DSS is somewhere behind the scenes, conjuring up the answers.
DSS is hardly a new thing. Depending on whom you ask, these data-crunching systems have been around since World War II, helping the Allies crack codes, or, at the very least, since the 1970s, when businesses began adopting computer systems more broadly.
It’s only with the “confluence of several forces” during the 21st century, Badinelli asserts, that DSS has become such a blistering area of attention recently. Those drivers include the development of cloud computing, which has disseminated data over a global network, making it accessible “to just about anybody”; the advent of mobility and the internet of things, which has connected “billions of devices” to networks and generated petabytes of data; and the explosion of social media, which has also generated “a wealth of micro-data that wasn’t available before.”
Cliff Ragsdale, also a professor at the Pamplin College of Business and academic director for Virginia Tech’s Center for Business Intelligence and Analytics, gives a formal explanation of DSS. DSS consists of five components: a computer system to process the data; modeling capabilities to emulate the decision-making process; domain-specific knowledge (such as all that medical literature); a user interface through which the decision-maker can interact with the computer system; and, most important, the user -- a professional with the skills and talents to extract the relevant, data-driven conclusions.
As Ragsdale emphasizes, the computer doesn’t have to be a Watson-caliber system to do the work; in fact, you probably possess the tools you need to do DSS work. (More on that shortly.)
What DSS produces is three kinds of modeling:
- Descriptive, which constructs a frame for the decision as a cause-effect relationship from the options available to the decision-maker to the outcomes of each potential choice.
- Predictive, which uses that backward-looking data to figure out what is most likely to happen in the future given similar conditions.
- Prescriptive, which describes how to position the response to accomplish a given mission -- or, as Ragsdale puts it, “How do we use what we expect to come to change the outcomes?”
While anybody in business is accustomed to the output of descriptive modeling (the usual quarterly report), and some organizations have adopted predictive modeling (the ramp-up of Tesla Model 3 production through the next 12 months), it’s the little-used prescriptive modeling that will soon take center stage as more people become formally educated on it.
DSS in the wild
That’s the kind of work that Ragsdale is immersed in as a consultant to the industry. For example, a project that Ragsdale led with a team of Virginia Tech students from the master’s in business analytics program involved a federal agency that grants money to various nonprofit groups to provide services to society. The agency was concerned, he recalls, with “allocating tax dollars to these entities in an efficient and objective way.” He and his team used historical data (descriptive analytics) to look at the effectiveness of the nonprofit groups as a function of how much money they received and how they could use it. That analysis allowed them to predict the level of utilization they would achieve for a given level of funding (predictive modeling). Since funding is never unlimited, however, the team went a step further and developed a DSS that would allow the agency to weight the nonprofit groups based on how critical their services were. The use of a predictive model now allows the agency to develop an initial budget allocation based on the outcomes they want to see for the coming year instead of “just doing what we did last year and using that as a starting point.”
The modeling tool Ragsdale used for each of those stages? Microsoft Excel -- the same tool he works with in his Virginia Tech online master’s courses on DSS, made up primarily of working professionals. He concedes that students aren’t necessarily impressed when they begin the course and find out they’ll be working with Excel. “I see the [virtual] eye rolls, and a lot of people will say they have 10 years of experience with Excel,” he notes. “But what they really have is six months of experience repeated 20 times.”
The magic of using Excel is the “ubiquity of the platform. On pretty much every computer you’ll find a copy of Excel or some spreadsheet.” By the time those students have finished the class, says Ragsdale, their common response is: “Wow! I had no idea Excel could do this.”
Badinelli takes a different approach. Although Watson may not be a standard application on every computer, it certainly has a presence in any industry with data and decision-making (in other words, every industry). So his course teaches students how to communicate with Watson through their own accounts on BlueMix, IBM’s slate of tools, including the Watson interface. Student teams pick a topic area and load relevant documents into the DSS to train Watson to answer various kinds of questions about that topic. For example, one team was interested in home oxygen machines used by people with illnesses or disabilities. Watson would pose as an assistant to advise them on how to set the machine for that day’s oxygen intake based on what activities the user had planned.
Employment Outlook
The job outlook for decision support managers is astonishing. The range of base salaries is $91,478-$120,022 with a median of $106,697, according to Salary.com. The median bonus for this position is $6,617 and the entire median compensation package, including salary, bonuses and benefits comes in at $154,759.
As you would expect, this role manages a team of decision support staff and acts as a liaison to upper management to share the outcomes of the modeling work. Salary.com recently listed 11,859 jobs with that or a similar title.
There’s a nuance here that’s important to point out. In this era of big data, warns Badinelli, “anybody with a statistics background and a warm pulse can call themselves a data scientist. Right now, it’s the hottest field going, and it will be for several years.”
Most of those people, however, are doing business analytics, an aspect of DSS, but not necessarily the most important one. As Badinelli explains, “"People are starting with data and asking, what can we do with the data?” The better approach is to “start with the decision and ask, what kind of data does this decision need?”
The DSS expert is better positioned to work with the decision-maker in exploring the big problems to be solved, the options available and the potential outcomes. That kind of expertise has staying power beyond the latest hype cycle.
Better decision-making that matters
Class work like that has made the master’s degree in IT the “most employable major in the college of business,” says Badinelli. “Typically, a student graduating in May will have three job offers by October of the preceding year -- some of them with signing bonuses.”
However, adds Ragsdale, this kind of work isn’t just about the money grab. “We’re a business school, so we’ve got to talk about the money. But anymore, the thinking is evolving about what business organizations need to achieve.” The philosophy of the “triple bottom line” with its three P’s (profit, people and planet) has begun permeating the industry. “Sustainable business endeavors really need to think about all three of those things, not just the profit. If you profit at the expense of people and planet, you’re not going to be in business for long.”
And the beauty of learning the ins and outs of decision support systems is that the outcome is application-agnostic. Says Ragsdale, the study of DSS “has universal applicability. In pretty much any discipline or endeavor, there are important decisions to be made.”
Written by SmartBrief Education